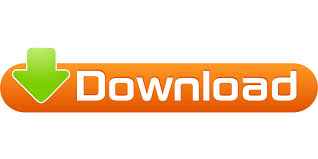
During the past few years, extensive efforts have been made towards the development of MLSFs, ranging from traditional ML-based approaches (e.g., RF-Score, NNScore, MIEC-SVM, Δ VinaRF 20, and AGL-Score ) to recently-emerged deep learning (DL)-based methods (e.g., AtomNet, DeepVS, K DEEP, and PotentialNet ), and most of these MLSFs demonstrate remarkably superior performance compared with the classical SFs. With the aid of state-of-the-art machine learning (ML) algorithms, MLSFs developed by automatically learning the generalized nonlinear functional forms from the training data have gradually emerged as a promising alternative to overcome the disadvantages of classical SFs. The former three can be collectively referred to as classical SFs, since all of them follow an additive formulated hypothesis to represent the relationship between the features that characterize protein–ligand interactions and experimental bioactivities. ĭepending on different theoretical principles, existing SFs can be typically divided into four main groups: physics-based, empirical, knowledge-based, and newly-emerged machine learning-based SFs (MLSFs). Despite impressive success of molecular docking, the deficiency of SFs remains a major obstacle to the reliability of real-world applications of docking. Typically, molecular docking has two stages: (1) sampling the pose of the ligand in the binding site of a macromolecular target (usually a protein) and (2) scoring the binding strength of the ligand to the target by using a predefined scoring function (SF). Molecular docking is one of the core technologies in structure-based drug design (SBDD), and it has contributed enormously to drug discovery and development in the past decades. We believe that our study may provide valuable guidance for the development and assessment of new machine learning-based SFs (MLSFs) for the predictions of protein–ligand binding poses.
#CODEJOCK DOCKING PANE FORCE DOCK CODE#
The source code and the newly-developed cross-docking datasets can be freely available at and, respectively, under an open-source license. Furthermore, our calculation results also highlight the importance of the incorporation of the cross-docked poses into the training of the SFs with wide application domain and high robustness for binding pose prediction. Besides, it is found that, despite the significant decrease of the performance for the threefold clustered cross-validation, the inclusion of the Vina energy terms can effectively ensure the lower limit of the performance of the models and thus improve their generalization capability. The calculation results illustrate that using Extended Connectivity Interaction Features (ECIF), Vina energy terms and docking pose ranks as the features can achieve the best performance, according to the validation through the random splitting or refined-core splitting and the testing on the re-docked or cross-docked poses. In this study, based on a cross-docking dataset dedicatedly constructed from the PDBbind database, we developed several XGBoost-trained classifiers to discriminate the near-native binding poses from decoys, and systematically assessed their performance with/without the involvement of the cross-docked poses in the training/test sets. Structure-based drug design depends on the detailed knowledge of the three-dimensional (3D) structures of protein–ligand binding complexes, but accurate prediction of ligand-binding poses is still a major challenge for molecular docking due to deficiency of scoring functions (SFs) and ignorance of protein flexibility upon ligand binding.
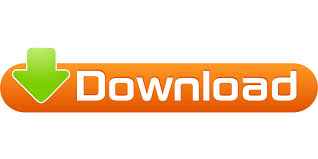